安装yellowbrick
pip install yellowbrick
代码
import pandas as pd
from sklearn.cluster import KMeans
from yellowbrick.cluster.elbow import kelbow_visualizer
datafile= r"../../ML_data/iris.csv"
data_df = pd.read_csv(datafile,header =0)
X = data_df[["sepal_length","sepal_width","petal_length","petal_width"]]
oz = kelbow_visualizer(KMeans(random_state=1), X, k=(2,10))
k = oz.elbow_value_
print(f"佳的K值是{k}")
print(f"elbow_score_值是{oz.elbow_score_}")
得到的拐肘图如下
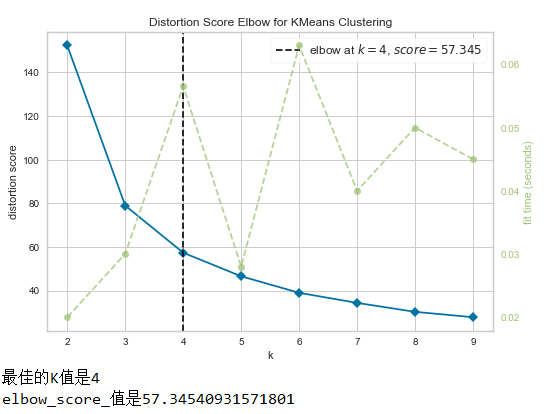
从图中可以看出佳的K值是4.
kelbow_visualizer的参数metric 表示度量每个点到其质心的距离之和的方法
metric : string, default: ``"distortion"``
Select the scoring metric to evaluate the clusters. The default is the
mean distortion, defined by the sum of squared distances between each
observation and its closest centroid. Other metrics include:
- **distortion**: mean sum of squared distances to centers
- **silhouette**: mean ratio of intra-cluster and nearest-cluster
distance
- **calinski_harabasz**: ratio of within to between cluster dispersion
分别用这三种度量方法
kelbow_visualizer(KMeans(random_state=1), X, k=(2,10),metric='distortion')
kelbow_visualizer(KMeans(random_state=1), X, k=(2,10),metric='silhouette')
kelbow_visualizer(KMeans(random_state=1), X, k=(2,10),metric='calinski_harabasz')
得到的拐肘图分别如下
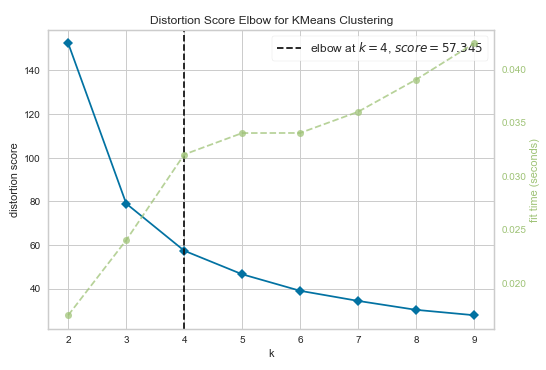
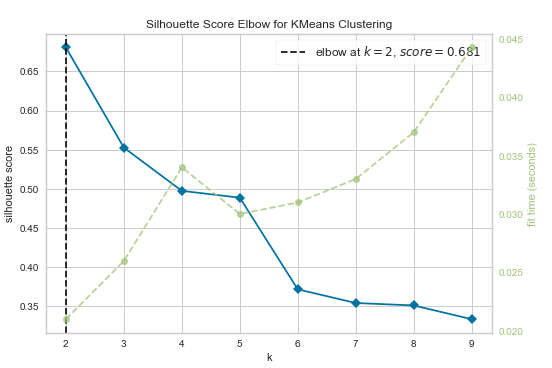
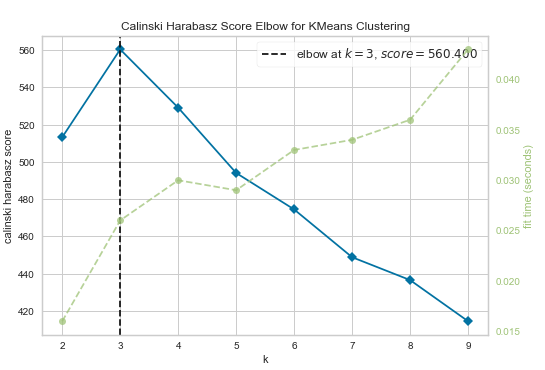
这三种度量方法得到的K值分别是4、4、3.
来源 https://zhuanlan.zhihu.com/p/396665902