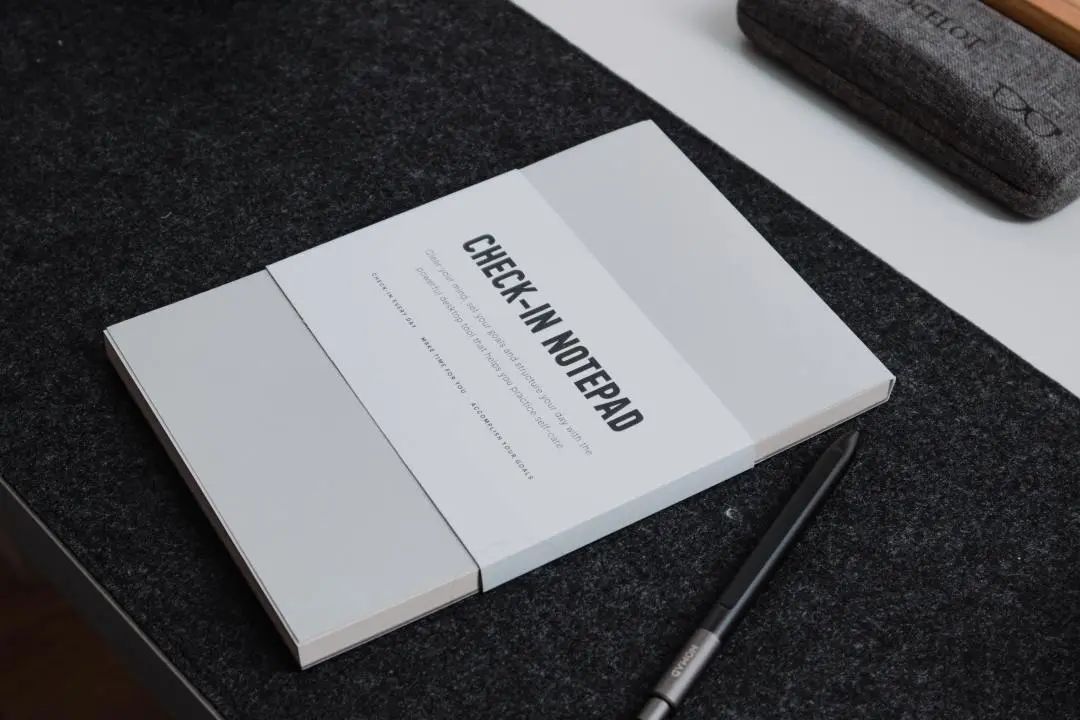
作者介绍
高文佳
2021年9月加入去哪儿网 DBA 团队,主要负责公司酒店业务和支付业务的数据库管理运维,具有多年数据库运维管理经验。
一、场景描述
某业务线在 Redis 中使用使用 HASH 对象来存放数据,并使用 HSCAN 命令来循环遍历 HASH 对象中所有元素,业务上线后平稳运行很长时间,但在某天 Redis 实例 QPS 较低(小于 1000)且无明显波动情况下,Redis 实例 CPU 使用率缓慢爆涨至 ,应用程序请求 Redis 的响应时间也明显增加导致业务异常。
Redis 实例 CPU 使用率监控:
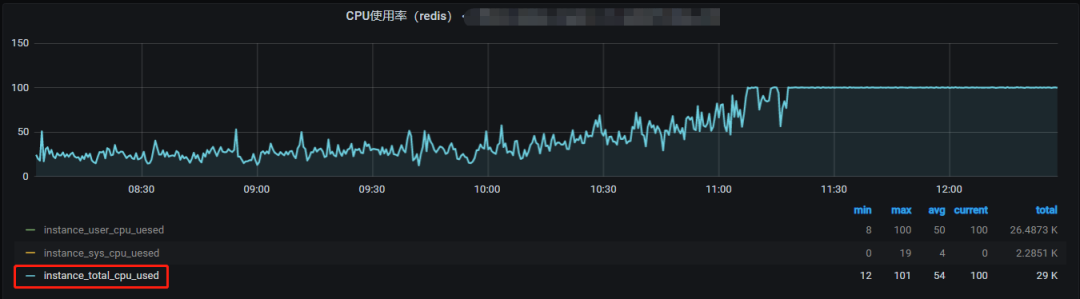
Redis 实例 QPS 监控:
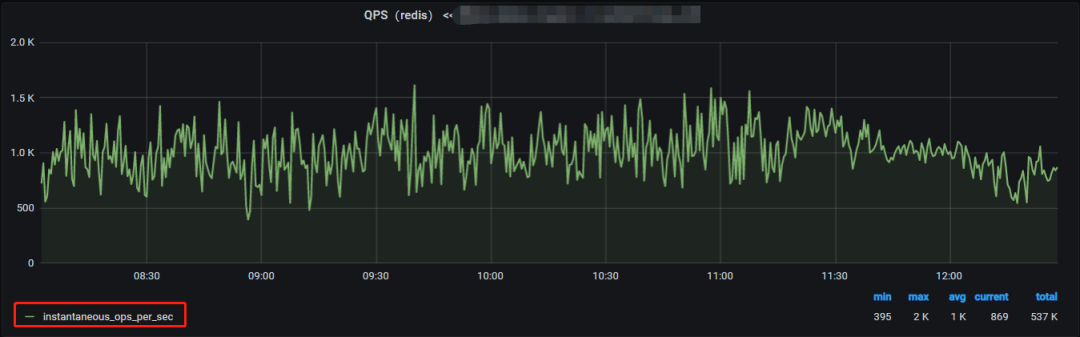
二、问题分析
通过 Redis 的慢日志和 Redis 命令耗时监控,我们快速定位到 Redis 实例 CPU 使用率较高的"元凶"就是命令 HSCAN XXX 0 COUNT 100,但为何 HSCAN 默认匹配所有元素且限制返回数量 100 的情况下还执行这么长时间?
通过官方文档可用找到如下描述:
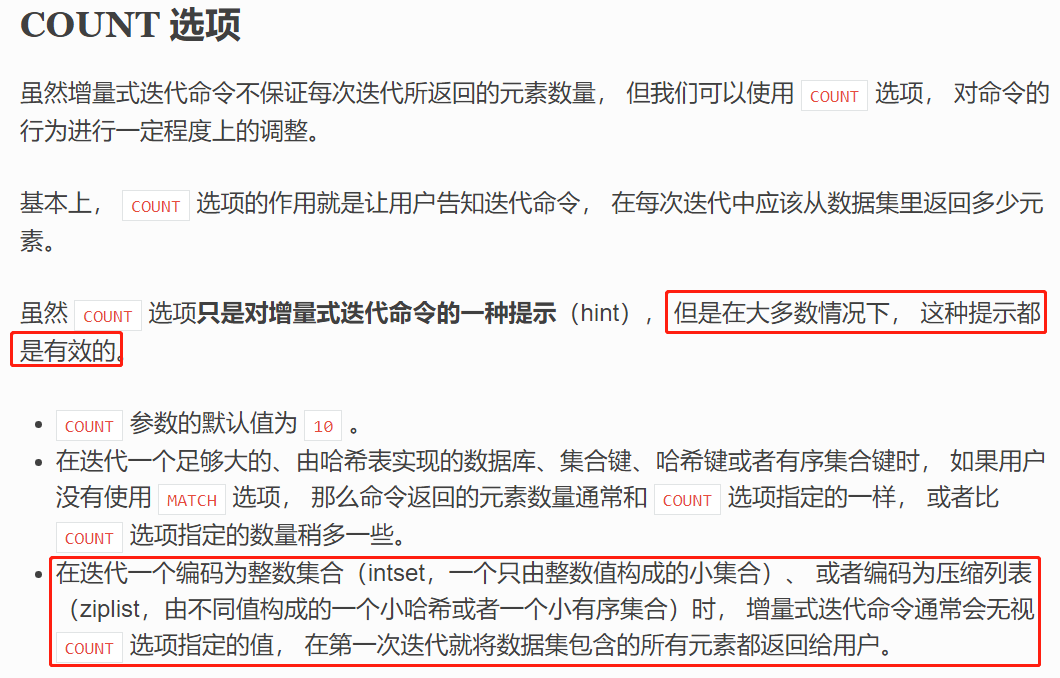
挑选其中一个 KEY 进行分析:
## 查看KEY的编码类型
redis 127.0.0.1:8662> DEBUG OBJECT "XXX_XXX_572761794"
Value at:0x7fd4aa9d73f0 refcount:1 encoding:ziplist serializedlength:27573 lru:3322719 lru_seconds_idle:103
## 查看KEY的元素个数
redis 127.0.0.1:8662> HLEN "XXX_XXX_572761794"
(integer) 1196
## 查看ziplist相关配置参数
redis 127.0.0.1:8662> CONFIG GET '*ziplist*'
1) "hash-max-ziplist-entries"
2) "2048"
3) "hash-max-ziplist-value"
4) "3072"
5) "list-max-ziplist-size"
6) "-2"
7) "zset-max-ziplist-entries"
8) "2048"
9) "zset-max-ziplist-value"
10) "3072"
根据当前Redis实例配置,当 HASH 对象的元素个数小于 hash-max-ziplist-entries (2048)个或 HASH 对象的元素值长度低于 hash-max-ziplist-value(3072)字节时会使用 ziplist 编码方式来存储 HASH 对象,而 HSCAN 命令对于编码类型为 ziplist 的集合类型会忽略 COUNT 参数值而全量返回所有元素,在这种场景下 HSCAN 性能较差。
三、源码学习
以 Redis 5.0 代码为例,使用 hscanCommand 函数来处理 HSCAN 命令,实际调用 scanGenericCommand 函数来处理:
void hscanCommand(client *c) {
robj *o;
unsigned long cursor;
if (parseScanCursorOrReply(c,c->argv[2],&cursor) == C_ERR) return;
if ((o = lookupKeyReadOrReply(c,c->argv[1],shared.emptyscan)) == NULL ||
checkType(c,o,OBJ_HASH)) return;
scanGenericCommand(c,o,cursor);
}
函数 scanGenericCommand 的代码实现共分为 4 个步骤:
- 步骤:解析参数(Parse options)
- 第二步骤:遍历集合(Iterate the collection)
- 第三步骤:过滤元素(Filter elements)
- 第四步骤:返回结果(Reply to the client)
在遍历集合的第二步骤,会根据集合的编码类型做相应处理:
- 如果编码类型为 HashTable 或 SkipList 时,会按照要返回元素数量(count)来设置大遍历次数(maxiterations= count *10 ),然后使用"返回元素数量"和"大遍历次数"双重限制下调用 dictScan 来遍历集合,确保 SCAN 操作不会遍历过多数据导致执行时间超过"预期"。
- 如果编码类型为 IntSet 时(数据类型为 Set 且编码类型不为 HashTable),会设置 cursor=0 并遍历整个 IntSet 集合。
- 如果编码类型为 ZipList 时(数据类型为 HASH 且编码类型不为 Hashtable,数据类型为 ZSet 且编码类型不为 SkipList),会设置 cursor=0 并遍历整个 ZipList 集合。
函数 scanGenericCommand 代码:
void scanGenericCommand(client *c, robj *o, unsigned long cursor) {
/* Step 1: Parse options. */
/* Step 2: Iterate the collection.
*
* Note that if the object is encoded with a ziplist, intset, or any other
* representation that is not a hash table, we are sure that it is also
* composed of a small number of elements. So to avoid taking state we
* just return everything inside the object in a single call, setting the
* cursor to zero to signal the end of the iteration. */
/* Handle the case of a hash table. */
if (ht) {
long maxiterations = count*10;
/* We pass two pointers to the callback: the list to which it will
* add new elements, and the object containing the dictionary so that
* it is possible to fetch more data in a type-dependent way. */
privdata[] = keys;
privdata[1] = o;
do {
cursor = dictScan(ht, cursor, scanCallback, NULL, privdata);
} while (cursor && maxiterations-- && listLength(keys) < (unsigned long)count);
} else if (o->type == OBJ_SET) {
int pos = ;
int64_t ll;
while(intsetGet(o->ptr,pos++,&ll))
listAddNodeTail(keys,createStringObjectFromLongLong(ll));
cursor = ;
} else if (o->type == OBJ_HASH || o->type == OBJ_ZSET) {
unsigned char *p = ziplistIndex(o->ptr,);
unsigned char *vstr;
unsigned int vlen;
long long vll;
while(p) {
ziplistGet(p,&vstr,&vlen,&vll);
listAddNodeTail(keys,
(vstr != NULL) ? createStringObject((char*)vstr,vlen) :
createStringObjectFromLongLong(vll));
p = ziplistNext(o->ptr,p);
}
cursor = ;
} else {
serverPanic("Not handled encoding in SCAN.");
}
/* Step 3: Filter elements. */
/* Step 4: Reply to the client. */
}
Redis 命令 HGETALL 也是全量返回所有元素,其实现逻辑则相对简单:
void genericHgetallCommand(client *c, int flags) {
robj *o;
hashTypeIterator *hi;
int multiplier = ;
int length, count = ;
if ((o = lookupKeyReadOrReply(c,c->argv[1],shared.emptymultibulk)) == NULL
|| checkType(c,o,OBJ_HASH)) return;
if (flags & OBJ_HASH_KEY) multiplier++;
if (flags & OBJ_HASH_VALUE) multiplier++;
length = hashTypeLength(o) * multiplier;
addReplyMultiBulkLen(c, length);
hi = hashTypeInitIterator(o);
while (hashTypeNext(hi) != C_ERR) {
if (flags & OBJ_HASH_KEY) {
addHashIteratorCursorToReply(c, hi, OBJ_HASH_KEY);
count++;
}
if (flags & OBJ_HASH_VALUE) {
addHashIteratorCursorToReply(c, hi, OBJ_HASH_VALUE);
count++;
}
}
hashTypeReleaseIterator(hi);
serverAssert(count == length);
}
四、性能对比
对于全量返回 HASH 对象所有元素 KEY 和 VALUE 的场景,HGETALL 和 HSCAN 那个性能好呢?
模拟创建一个包含 2000 个元素的 HASH 对象:
def init_hash_key():
"""
初始化一个包含2000个元素的HASH对象
"""
hash_key = "test_hash_01"
hash_item_count=2000
redis_conn = redis.Redis(connection_pool=redis_pool)
redis_conn.delete(hash_key)
for item_index in range(, hash_item_count):
hash_item_key = "test_42764865253_42764865253_572774616_" + str(item_index)
hash_item_val = """ {"roomId":"42764865253","productId":507346697,"lastDate":"20220316","index":" """ \
+ str(item_index) + """ "} """
redis_conn.hset(
name=hash_key,
key=hash_item_key,
value=hash_item_val
)
pass
然后采用10个进程(multiprocessing.Process)来进行 HGETALL 和 HSCAN 的测试:
def loop_hscan(loop_times=10000):
hash_key = "test_hash_01"
redis_conn = redis.Redis(connection_pool=redis_pool)
for run_index in range(, loop_times):
redis_conn.hscan(name=hash_key, cursor=, count=100, match="*")
def loop_hgeall(loop_times=10000):
hash_key = "test_hash_01"
redis_conn = redis.Redis(connection_pool=redis_pool)
for run_index in range(, loop_times):
redis_conn.hgetall(hash_key)
Redis 实例 CPU 使用率监控:
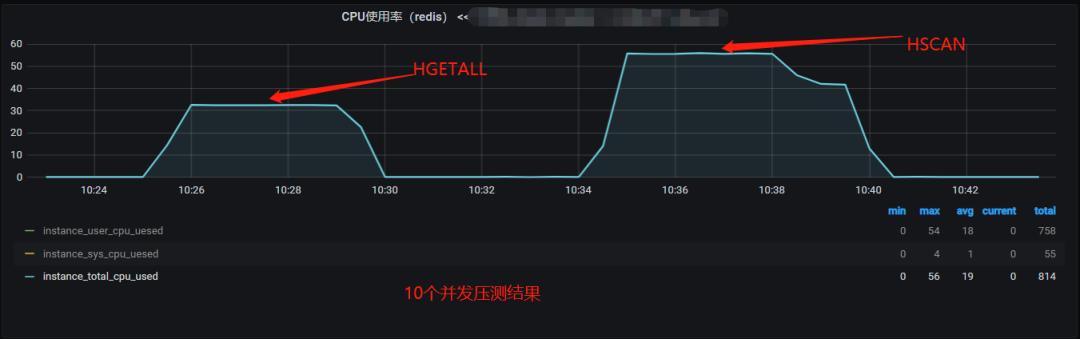
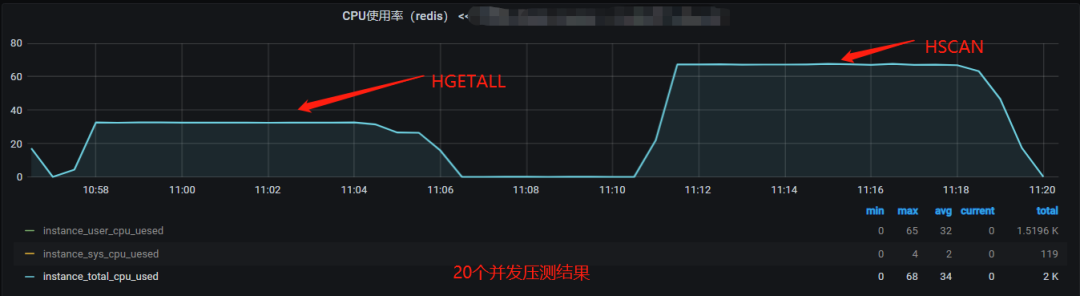
Redis 实例 QPS 监控:
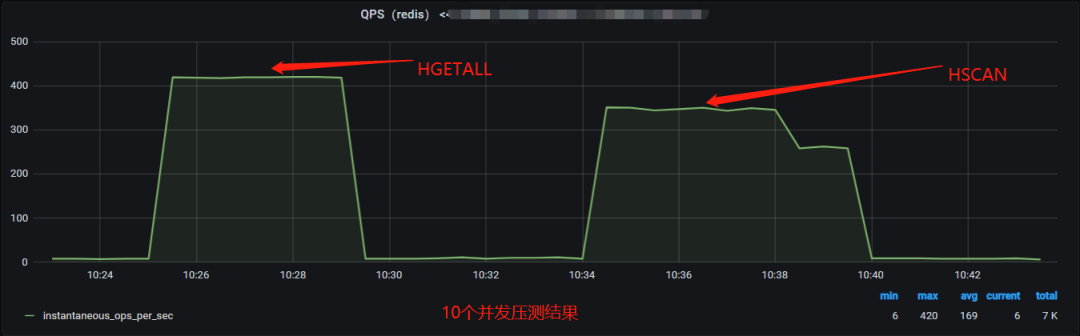
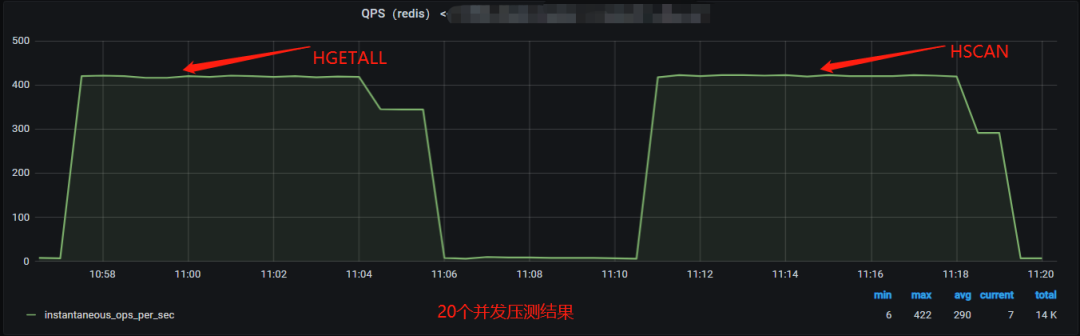
压测服务器网络流量监控:
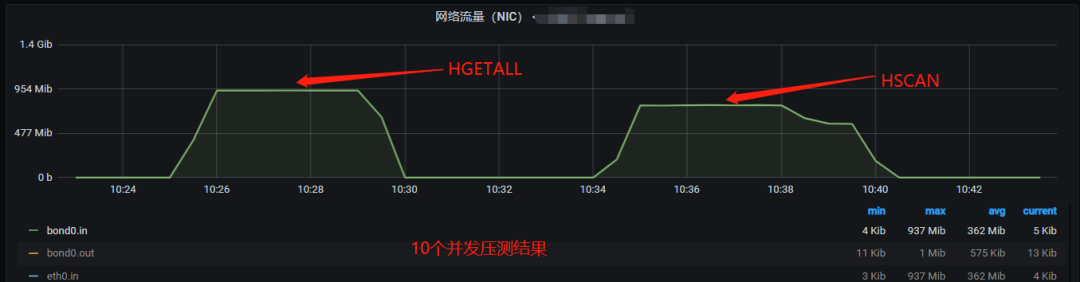
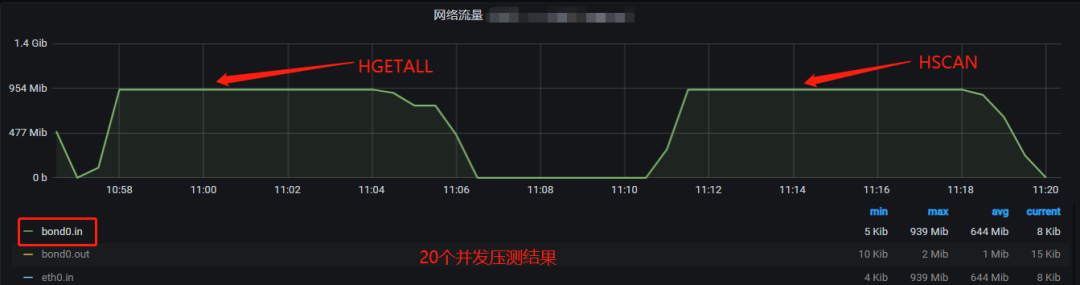
受限于测试服务器的千兆网卡限制,HGETALL 和 HSCAN 的测试高 QPS 达到 410。
对比 10 个并发的压测结果可发现:
对于单次请求操作的请求耗时,HGETALL 命令远低于 HSCAN 命令。
对比 20 个并发压测结果可发现:
对于相同 QPS 产生的 Redis 服务器网络带宽,HGETALL 命令和 HSCAN 命令基本相同(因为返回的数据基本相同)。
对于相同 QPS 产生的 Redis 服务器 CPU 资源,HGETALL 命令(33%)约占HSCAN命令(67%)的一半。
五、优化建议
对于编码类型为 IntSet 和 ZipList 的 Redis 集合对象,在执行 HSCAN 命令是会忽略 COUNT 参数并遍历所有元素,当 Redis 集合对象元素较多时会严重消耗 Redis 服务器的 CPU 资源,对于需要全量返回所有元素的场景,可以使用 HGETALL 命令替换 HSCAN 命令,但对于需要过滤部分元素的场景,HSCAN 命令能在 Redis 服务器端进行过滤以降低应用服务器和 Redis 服务器之间的网络带宽消耗。
虽然可以通过参数来控制 Redis 各种类型的编码方式,将 Redis 集合对象的编码类型从 IntSet 和 ZipList 转换为 HshTable 或 SkipList,以避免 HSCAN 命令全量扫描集合对象的所有元素,建议谨慎调整此类参数设置避免引发其他如内存使用率上涨等问题。
在实际业务场景中应尽量避免使用 HSCAN 和 HGETALL 等命令,尤其是使用这些命令操作超大 Redis 集合对象并高频执行,应从业务角度评估其实现合理性,并通过改写业务逻辑/增加前端缓存/使用数据压缩等方式来降低 Redis 服务器端请求压力。
原文链接:https://mp.weixin.qq.com/s/sdG7R2_0WYVnWTZ9arAACw